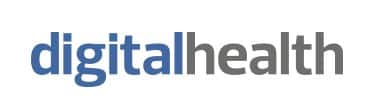
Special Report: AI and Data
In the post-pandemic world, the onus seems to be on artificial intelligence (AI) to carry the healthcare sector forward. Maja Dragovic finds out how the attitudes towards AI in the sector have changed over the last 12 months.
The focus on AI has definitely shifted since the pandemic, with data being seen as a tool to improve the health and care of a population in a safe, trusted and transparent way.
The government’s recent data strategy for health and care has set the direction for the use of data in a post-pandemic healthcare system with AI playing a significant role, especially in screening services where the technology can help scan numerous hospital images for irregularities.
For Chris Scarisbrick, sales director at Sectra, an accelerator of AI use in screening was the development of the National Lung Screening pilot. This involved various vendors being bought in to help meet the increased demand of chest X-rays during the pandemic. However, it transpired that in the long run, using different AI providers resulted in integration and procurement challenges for healthcare providers.
Scarisbrick points out that “at any given time, there might be 50 or 100 startups in the AI space”.
“If an NHS organisation identifies 10 or 15 different use cases where the AI could be brought in to help alleviate their problems, then they have a massive problem in terms of procuring and contracting that number of applications,” he says.
There are numerous issues connected with this, Scarisbrick adds, and that includes the deployment of these applications – would they be deployed on NHS infrastructure, or can they be deployed in remote cloud environments; who will be responsible for information governance; will data be safe and so on.
To address these issues, Sectra has developed a marketplace concept through its amplifier store where, rather than have an NHS Trust procure and contract with the 10 or 15 different applications that they need to engage with, the company can handle that side of things.
“We’ll take on that burden for them, we will do the contracting upfront, and then we will present it back to the customer through our application store so that they can pick and choose which algorithms they want to consume,” Scarisbrick says.
A useful toolset
While screening is a massive segment of AI use, its adoption is spilling over to other areas, too. AI is seen as a tool which can help the NHS predict where emergency services are most likely to be needed which in turn allow trusts to plan accordingly. It has been touted as a tool which can help with hospital bottlenecks such as waiting lists.
Oracle Cerner has developed an elective recovery dashboard, which links information from electronic health records and patient treatment lists to help NHS organisations identify patients and cohorts for prioritisation and intervention.
“At the moment, the tool provides system-wide analytics with an ability to break down information by different geographic areas and the characteristics of the patients such as demographics,” explains Jurgita Kaubryte, the company’s senior data scientist for population health across Europe.
The software provider is also working on developing a risk stratification model that can stratify those patients based on the risk of an adverse event which will help prioritise the patients on the waiting list using AI.
Analytics platform is a must
Looking at the analytics side of AI, Scarisbrick argues for the importance of measuring the performance of AI to be able to answer questions such as: is this technology for technology’s sake, or is this providing real world clinical value?
“The early adopters that are putting AI out there today, if they can bring to the market some very clear analytics behind the decisions and the efficacy that the AI is bringing to their institutions, then that’s a really solid proof point for other organisations within the NHS to learn from and adopt for themselves moving forward,” he adds.
Implementing analytics tools for AI doesn’t have to mean starting from scratch, however. Orion Health has developed a maturity model that enables customers to iteratively build out from existing shared care records to add functionality for analytics and further tools to support population health management. They believe that this is where NHS focus will be in the near term, before attention turns to incorporating AI and machine learning in the future.
In terms of machine learning, the company continues to drive research as part of New Zealand’s Precision Driven Health Partnership and has delivered an algorithm hub on a national scale in the country.
“The Hub uses Orion Health’s Machine Learning Manager to store and administer a series of algorithms that are publicly accessible, including for the district health boards as they’re known in New Zealand, similar to ICS’s in England,” explains Bruce Horne, the company’s product specialist Lead in the UK and Ireland.
The Hub has been live in New Zealand for over a year, initially coming about as part of the country’s response to the Covid-19 pandemic.
“The New Zealand government wanted to make these algorithms, such as risk of readmission, publicly available to enable the Ministry of Health and district health boards to better care for their populations,” Horne says.
“As an example of machine learning in action, the Waitematā District Health Board (WDHB) is currently using a machine learning model that predicts a patient’s readmission risk from their second day in hospital. Risk of readmission scores are calculated for patients being discharged daily and surfaced in an analytics dashboard for reporting and planning purposes. In the future, WDHB will look to leverage this score for effective discharge planning and to avoid preventable readmissions, and to build it into clinical workflow.”
Moving from reactive analytics to preventative use
Due to its capability to scan huge amounts of data, AI is being deployed to tackle population health.
With Integrated Care Systems (ICSs) being formed across England, their focus will not only be on the acute events, but events happening across the whole region, says Ben Court, head of analytics at Civica. This will allow for AI to look at prediction and preventative measures.
The structures to deploy this are already in place, adds Court, since there were lots of practical data sharing programmes that occurred through Covid-19, where data was shared between GPs, social care and acute settings.
What has also become apparent in the process, continues Court, is the possibility to share best practice examples in care provision.
“If a clinician in a hospital is doing really well, and doing it at a cost-effective way, what does he know that other people don’t know? Does he have access to equipment? Does he have a process? Does he work in a particular way that can be shared? So we’re starting to look at ways that we can share best practice by identifying variations in healthcare cost, which is linked to healthcare delivery,” Court concludes.
One size does not fit all
But what works in one area might not be applicable in another, Manuel Reyes, Cognizant’s chief architect points out.
“Could a dataset from a GP practice in a very affluent area of central London be modelled in a way that would be suitable to provide care for somebody who lives in the Orkney Islands? Probably not,” he says.
This is a simple bias, Reyes says, adding that there are societal biases around different demographics, and different people with different backgrounds that also need to be taken into consideration.
“There are certain communities that engage quite heavily with primary care, for example and there are other communities that don’t,” he adds.
“We saw that in Covid, there were certain communities that were more open to testing and to vaccination. But there were other communities that for their own reasons were not. So it’s about understanding the biases that exist in the data as well.”
There are other challenges that will need to be tackled to ensure the optimum use of AI, not least the lack of national integration standards. This means that healthcare organisations have manipulated integration models such as ADT a28 messages to fit their local needs, says Reyes.
“This was great, because it’s local problems being solved in local ways. But when you try and bring that data together, you’ve now got an integration challenge, because you’ve got to try and aggregate that data from up to 250 systems,” he adds.
Lack of standards is also reflected in the way that health and care providers store their information, resulting in huge amounts of unstructured data, says Reyes. And AI needs structured data. While imaging is an example of best forms of structured data, other areas are still seeing a challenge because there is a lack of “single canonical standards”.
What about the risk? According to Peter Tyreholt, chief product officer for Visiba, with healthcare sector being very risk adverse, challenges will need to be tackled as providers would still rather opt to do nothing, than risk making a mistake, especially when it comes to AI.
Personalised care
What is certain, however, is that AI will play a huge role in personalised care in the future.
“AI will enable patients to be given treatments which are based on their specific clinical and demographic characteristics, rather than a one size fits all approach,” Oracle Cerner’s Kaubryte concludes.
“AI and data science techniques can quickly process huge amounts of data about the patient and systematically compare that information with the data from similar patient cohorts and available scientific evidence, which can then help inform clinical decisions.”
[adbutler zone_id=”575582″]