Advisory Series: Predictive Analytics
- 26 September 2018
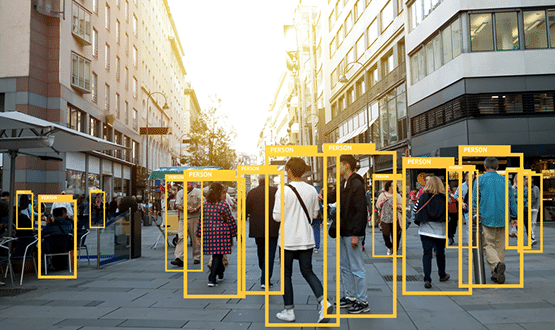
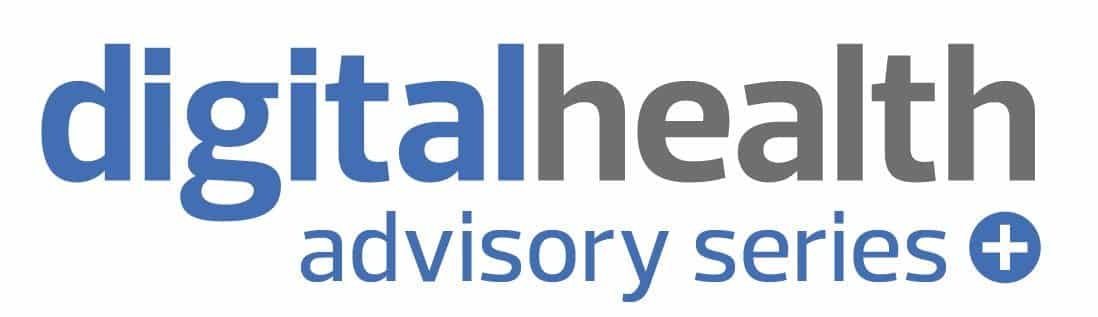
Predictive Analytics – Advisory Series, September 2018
By Vivienne Raper – Digital Health
When looking at the promise of predictive analytics in healthcare – namely, the ability to identify a downturn in someone’s health and intervene before it becomes more serious – the focus has often been on physical healthcare. But, as Vivienne Raper reports, more and more organisations are exploring the role such data analysis could play in supporting those with mental ill health.
In her position as research manager at the Mental Health Foundation, Josefien Breedvelt frequently encounters conversations on how data analysis could lead to improved care. “There’s a lot of discussion about using predictive analytics in mental health for better decision making and outcomes,” she says. “It can definitely be very positive for the NHS and local services, but a lot of work still has to be done.”
Predictive analytics involves using existing data to predict future outcomes and, in healthcare, the idea is to identify high risk patients. If they can be spotted early, the reasoning goes, then intervention can also be put in place early – leading to a better outcome for the individual, and reduced costs for the health service.
“There’s quite a history of applying these methods for people with physical health conditions, with examples going back a decade or so, but it’s quite a new thing to use these algorithms to see which patients are at risk of a mental health crisis,” says Adam Steventon, director of data analytics at the Health Foundation.
A national model
In Scotland, an algorithm to assess the risk of emergency inpatient admission has been in use since 2006. Scottish Patients at Risk of Readmission and Admission (SPARRA) calculates risk scores for around 4.2 million patients in Scotland aged 16 and above.
Upgraded to Version 3.0 in 2012, SPARRA today bundles mental health patients into long term conditions, and includes an algorithmic component covering their risk of admission to hospital. Initially targeted at GPs, the intention of SPARRA is to alert healthcare professionals that a patient may need to be called in for a review.
Predictive analytics in England
Over the border in England, at least two NHS trusts are beginning to apply predictive analytics to identifying high risk mental health patients. Birmingham and Solihull Mental Health NHS Foundation Trust is at the exploratory research stage of a NHS test bed programme designed to improve mental health urgent care. It will use predictive analytics to assess when patients may enter crisis.
Meanwhile Mersey Care NHS Foundation Trust, like Birmingham and Solihull a global digital exemplar, finished a separate pilot study in June this year. Working with a private sector partner, five years’ worth of data was analysed to predict the risk of someone moving into mental health crisis.
“That crisis could be crash landing in A&E, moving to an inpatient bed, or it could be self-harm, suicide or an escalation into serious depression,” explains Jim Hughes, director of informatics and performance improvement at the trust.
The pilot was established in two of the trust’s community mental health hubs. The algorithm had a dashboard-style presentation, showing metrics including numbers of people per caseload, as well as a box indicating patients at different levels of risk.
“The way it’s used was to provide additional information for multidisciplinary team meetings,” explains Hughes. The teams could use the algorithm to “dig down and look at an individual, to discuss who’s recently seen them, whether there’s anything in their life not recorded yet, and whether there’s a need to make an intervention such as a visit. So what’s happening is clinical decisions are enhanced by the tool”.
Hub managers could also use the tool to manage caseloads, by seeing whether any of their team were managing more patients at risk.
The project is paused while the trust shifts to a new electronic patient record. But once that move is complete, the plan is to reintroduce the analytics product to the hubs that previously used it – and to extend it into the rest of the organisation’s community teams.
The trust is running two other projects on predictive analytics and mental health crisis. One involves applying natural language processing and machine learning to clinical therapy notes and other text. “So it’s another way to stratify patients, but also to summarise many years of notes for an individual,” says Hughes.
Predicting suicide
In the USA, the picture on predictive analytics in mental health is similar to England. “I would say it’s becoming more common, but it’s not yet commonplace,” says Gregory E. Simon, a senior investigator at the Kaiser Permanente Washington Health Research Institute and psychiatrist for the Washington Permanente Medical Group.
Simon has been using predictive analytics to assess suicide risk. He led a study, published this year, to create an analytics model to predict suicide risk in the 90 days following a mental health visit.
“We’re getting ready to roll out in the Washington region of Kaiser Permanente and other regions will follow,” he explains.
Seizing the digital agenda
Joe Rafferty sees such work as a paradigm shift. “At the moment, I’d say our system – the NHS system generally – is see and treat,” says the chief executive of Mersey Care NHS Foundation Trust. He hopes analytics will afford the opportunity to move to “predict and prevent”.
If someone with existing illness has, for example, a relationship break down, a debt problem, and potential homelessness – “that drives our algorithm really, really powerfully,” he says. “It gives us an opportunity to get a much more comprehensive package around them before they get too ill.”
Rafferty believes big data will help augment – rather than replace – clinical judgement. “You can probably only hold four to five key issues in your head at one time, but the complexity around some people’s mental illness means there’s often 30-40 issues in play,” he says.
Limits on models
Yet, despite the potential, predictive analytics has limits. “Any model is only as good as the data going into it,” argues Stephen Riddell, principal information analyst at NHS Information Services Division Scotland (ISD).
“You have to be careful how you implement these algorithms,” adds the Health Foundation’s Steventon. “They’re not always completely accurate, they do miss people at risk, and they may also identify people as high risk who aren’t.”
“Predictive models are built to predict, not to explain,” says Kaiser Permanente’s Simon. “People are already at high risk, so any causal explanation is foolish.” For example, if a model shows getting a certain prescription is a factor in suicide risk, that drug doesn’t necessarily cause suicides.
Models shouldn’t be used beyond the care settings they were designed for, he contends. “If we’re building a model to predict risk after a mental health speciality visit, we’re developing a model for that occasion,” says Simon. “If we build a model for a primary care visit, it’s a different model.”
And applying predictive models to physical health shows “it’s one thing to identify people at risk, but it’s not necessarily obvious to clinical teams or doctors what to do with the information,” says Steventon. “That’s tended to be where a lot of these algorithms fall down.”
The human factor
In 2014, the Samaritans generated controversy with a Twitter app designed to alert people that their followers may be depressed – based on the content of their tweets. But privacy isn’t the only challenge as mental health services embrace big data and digital. Poverty can be a factor in mental illness and some fear patients can be digitally excluded from projects that rely on the latest smartphone.
Rafferty also says cultural change within the NHS will be important, as “a lot of staff were trained in the analogue world”. Mersey Care, he explains, has 70-80 clinicians who act as digital leaders, meeting monthly to discuss where to use digital – as well as sharing concerns.
But the biggest barrier may be economics. For early adopters, digital transformations can potentially be done at less than full cost, because companies are interested in exploring the technology. “So there’s a general question as all of this matures, what will be the cost of entry around some of this?” says Rafferty.
Enhanced Understanding
By InterSystems
It’s well documented that people are living longer, and the citizens aged 65+ will absorb the greater portion of health and care resources. Increased age and multiple long-term conditions is the new normal. As a result, healthcare is in the process of a massive transition – patient demographics, delivery models, provider relationships, technology and consumer expectations are all changing.
With this transition well underway and the numerous interactions citizens now have with the health and care service, you have an enormous pool of data in every imaginable format, both structured and unstructured. To manage through changing care delivery and the overall health of your population, you need information and insight.
InterSystems HealthShare Health Insight brings all this data together, organises and normalises it, and then delivers it in near real-time to an extensible hypercube for reporting and analysis within the HealthShare environment. That same, cleansed, comprehensive data can also be loaded into purchased or self-developed analytics applications, enterprise data warehouses, or clinical decision support solutions to enhance understanding and maximise value throughout your healthcare enterprise.
Closed-Loop Care
Safe, coordinated care requires analytics delivered to clinicians in time to identify opportunities for action and enable interventions. This is the kind of closed-loop management that leads to reduced readmissions, improved sepsis rates, and lower per member per month costs for chronic disease populations. Health Insight makes closed-loop management possible through automated event logic and notifications configured for the issues that matter to you and your organisation. It enables you to properly manage those who are sick, and encourage healthy behaviours for those who are not.
Analytics to power healthcare transformation
Health Insight contains a complete analytics data model, infrastructure, and starter sets to add meaningful health analytics to our HealthShare Information Exchange product, power your own solutions, or serve up current, credible data to optimise other analytics investments. It includes:
Extensible Hypercube
The comprehensive health analytics data model is automatically populated in near real-time from HealthShare Information Exchange. Health Insight’s customisation framework lets you extend the model to include other sources and data types.
Analytics Builder
Information becomes accessible when delivered to decision makers in meaningful formats. Health Insight provides the tools to create fully interactive dashboards, reports, statistical and text analyses, and business graphics with high-level summaries and detailed drill downs. A starter set of dashboards provides examples to simplify the process of creating your own.
Data Management Application Programming Interfaces (APIs)
The Health Insight toolset lets you develop analytics applications using the data in HealthShare Information Exchange. It also includes a library of APIs for populating the Health Insight Hypercube from other data sources. As with all HealthShare solutions, Health Insight understands and supports all relevant healthcare interoperability standards.
Data Provisioning
In addition to its own rich analytics environment, Health Insight can provide data services to the rest of your analytics portfolio. These include conditioning, harmonisation, normalisation, and transport services to feed current, comprehensive and credible data to other applications.
Active Interventions
Static reports do little to change behaviour. Health Insight includes the logic and notification mechanisms to deliver analytics insights within clinical and business workflows for decision-making and true closed-loop care process management.
![]() |
|
Contact number: 017 5382 9647 Email: Erica.Bennett@InterSystems.com Our website: www.intersystems.com/uk/industries/health-and-care/nhs/ Twitter: @InterSystemsUK LinkedIn: www.linkedin.com/company/intersystems-uk/ Facebook: www.facebook.com/InterSystems/ Further reading: Predict the future, one data point at a time HBI Solutions Predictive Analytics [ssba]
|
Predictive analytics can revolutionise the effectiveness and efficiency of healthcare
By Prof. Matthew Cooke – Chief Clinical Officer, Capgemini UK
Charlie de Montfort – Analytics Consultant, Capgemini Invent
Predictive analytics is now widely used to improve services across a range of sectors as the increasing ability to handle large amounts of data has meant that predictions are more accurate. For example, the Met Office receives 215 billion data observations every day requiring huge computational capability; in turn, this has led to unprecedented forecasting accuracy.
At Capgemini, we have applied predictive analytics across several sectors: from working with retail banks to enable the accurate identification of customers at risk of defaulting on loans, through developing a tool to help water companies detect pipe leakage to predicting demand and therefore stock requirements in the retail sector. But examples in healthcare are rare. One of the notable exceptions is ambulance services that have been using predictive analytics for many years to predict the location of 999 emergency calls, to move ambulances to standby points where calls are most likely to occur, and to meet predicted dynamic demand rather than being based in ambulance stations.
The primary aim of healthcare systems is to reduce disease burden, and so the NHS must harness the power of innovative technologies, such as predictive analytics, to improve its operational efficiency. Many new sources of data exist including sensors, apps and trackers that are either worn or in the patient’s home. Data is now being integrated around the patient from multiple channels and is also measured less intermittently (e.g. at a clinic) and more continuously (e.g. a worn device) allowing more accurate time-series predictions.
Many factors affect patient demand on health services. For example, attendances at emergency departments can vary according to weather, local events, holidays, seasons, as well as by time of the week. But most research has taken a unifactorial approach. Healthcare is a complex adaptive system and predictive analysis needs to understand the interactions of multiple factors. This is now possible but very rarely undertaken. Part of the reason for the reluctance to undertake this analysis is that a system must also be able to respond to the predicted changes in demand. In the NHS there is a perception that this cannot be achieved, even though many other sectors are doing so. For example, we are only recently seeing the use of digital solutions that allow staffing levels to be dynamically adapted to levels of predicted demand.
Using data to understand and predict patient demand is an area of tremendous opportunity. Capgemini’s ‘Humanising Healthcare’ is a pioneering data-driven improvement methodology, which provides health commissioners and providers with an intelligent framework for understanding who their patients are, which services they use and what they cost. By linking patients using time-series data with their activity, cost, demographic profiles, ‘Humanising Healthcare’ applies advanced predictive techniques to segment the patient base and identify factors that predicate high consumers. This helps to prevent or lessen intensive consumption and release much need capacity.
Using predictive analytics in such a way will help the NHS achieve ‘The Triple Aim’: improving quality, improving the health of the population and achieving value and financial stability.
![]() |
|
Contact number: 078 7110 5505 Email: matthew.w.cooke@capgemini.com Our website: www.capgemini.com/gb-en/service/healthcare-life-sciences/healthcare/ Twitter: @CapgeminiUK LinkedIn: www.linkedin.com/company/capgemini/ Further reading: ‘Play Your Cards Right’ with delayed transfers of care No accidental emergency: what studying A&E reveals about ‘rising demand’ [ssba]
|
1 Comments
Even though algorithms can weight even hundreds/thousands of factors only a small number of these will be relevant in a particular crisis at a particular time. Making sure the algorithm can tell the user which factors carry what weighting can help modify specific problems and identify false positives and false negatives.
Comments are closed.