‘Less than one percent’ of diagnostic AI studies based on high-quality data
- 1 October 2019
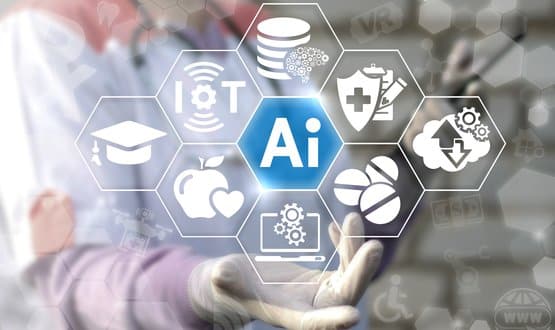
Less than one percent of available studies on the effectiveness of artificial intelligence (AI) in detecting diseases is supported by high-quality data, according to new research.
A comprehensive review of scientific literature led by University of Birmingham and University Hospitals Birmingham NHS Foundation Trust found that only a handful could be considered robust enough to back up their claims.
It suggested that many studies were biased in favour of machine-learning and tended to over-hype the ability of computer algorithms when comparing them to those of human healthcare professionals.
It consequently found that AI was able to detect diseases from medical images with a similar level of accuracy as healthcare professionals – contrary to several studies that have suggested AI can greatly outstrip human diagnosis.
The study concluded that, while machine learning held promise to aid clinical diagnosis, its true potential remained uncertain, and called for higher standards of research and reporting to improve future evaluations.
The research was described as “the first systematic review and meta-analysis synthesising all the available evidence from scientific literature”.
Published in the Lancet Digital Health, it involved reviewing over 20,500 articles published between January 2012 and June 2019 that compared the performance of deep learning models and health professionals in detecting diseases from medical imaging.
Of these, less than one percent were deemed “sufficiently robust in their design” and reported that independent reviewers had a high degree of confidence in their claims.
Further, only 25 studies validated the AI models externally using medical images from a different population, meanwhile just 14 studies used the same test sample to compare the performance of AI and health professionals.
Analysis of data from these 14 studies found that, at best, deep learning algorithms could correctly detect disease in 87% of cases, compared to 86% achieved by healthcare professionals.
The ability to identify patients who didn’t have disease was also similar for deep learning algorithms (93% specificity) compared to healthcare professionals (91%).
“Within those handful of high-quality studies, we found that deep learning could indeed detect diseases ranging from cancers to eye diseases as accurately as health professionals. But it’s important to note that AI did not substantially out-perform human diagnosis,” said Professor Alastair Denniston, University Hospitals Birmingham NHS Foundation Trust.
The authors also highlighted limitations in the methodology and reporting of AI-diagnostic studies included in the analysis, noting that deep learning was “frequently assessed in isolation in a way that does not reflect clinical practice.”
For example, only four studies provided health professionals with additional clinical information that they would normally use to form a diagnosis in a real-world setting.
Few of the studies were performed in a real clinical environment, and poor reporting was common, with most studies not reporting missing data, which the researchers noted would limit the conclusions that could be drawn from them.
A key lesson
Dr Xiaoxuan Liu, of the University of Birmingham, added: “There is an inherent tension between the desire to use new, potentially life-saving diagnostics and the imperative to develop high-quality evidence in a way that can benefit patients and health systems in clinical practice.
“A key lesson from our work is that in AI – as with any other part of healthcare – good study design matters. Without it, you can easily introduce bias which skews your results.
“These biases can lead to exaggerated claims of good performance for AI tools which do not translate into the real world. Good design and reporting of these studies is a key part of ensuring that the AI interventions that come through to patients are safe and effective.”
1 Comments
Quel surprise!
Comments are closed.